Bridging the Data Culture Gap: A New Framework
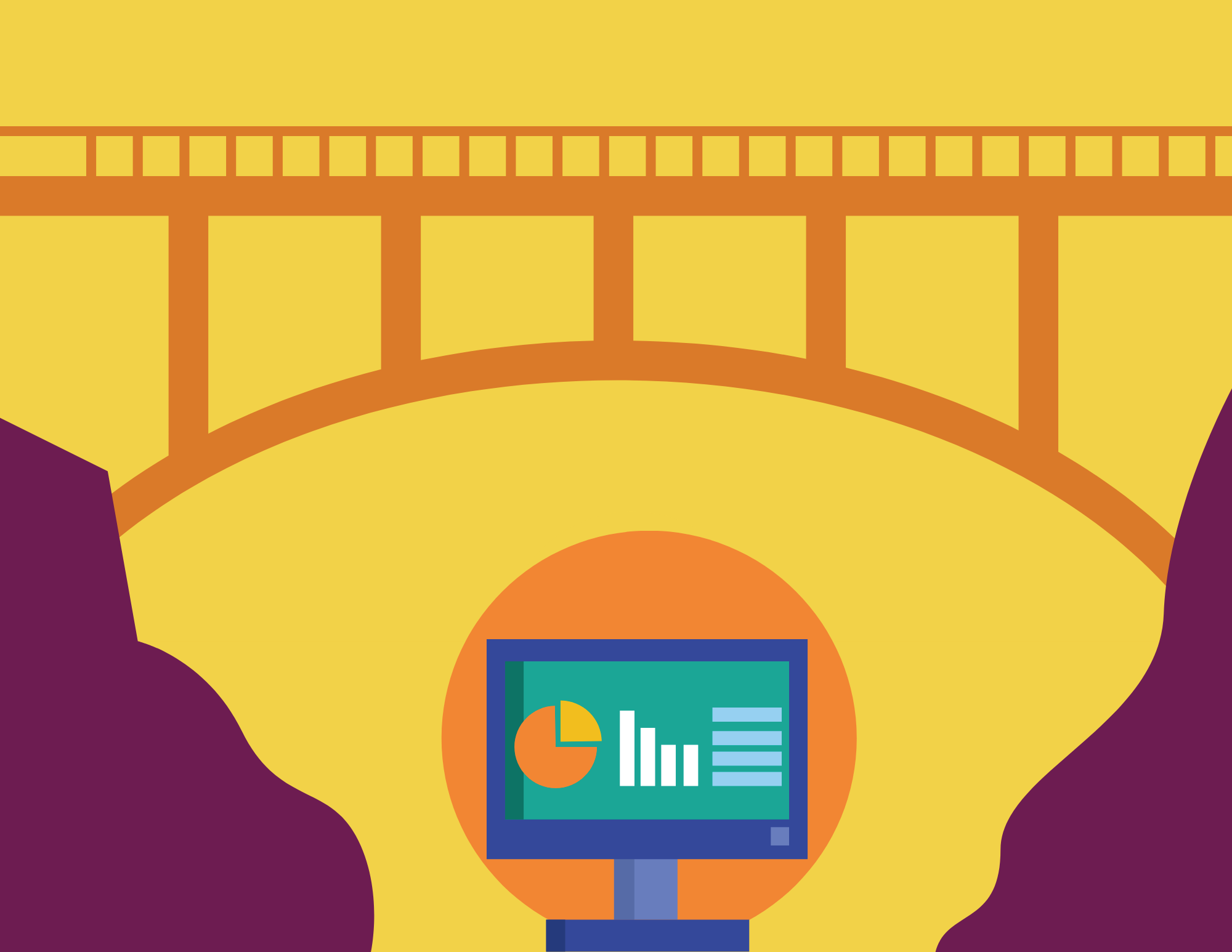
With the emergence of data science as a critical driver of business decisions, an ongoing debate has emerged around how to apply data science more effectively as a delineator of information.
The distance between the skills of data scientists and the needs of data users is sometimes called the “Data Culture Gap.” The question often posed at the center of this discussion is this: “How can data scientists resolve difficult problems and work more productively with data users?” But is this really the right question to ask?
Saran Ahluwalia, a Digital Services Expert and Data Scientist in the U.S. Digital Service, and a data scientist, believes it is time to reframe the discussion with different questions and criteria.
He says organizations like CMS should take a broader and more enlightened view of data-driven analysis and decision-making. In the following interview, Saran discusses alternate ways that data scientists, data users, and CMS leaders can work together to more effectively resolve problems and extract insights that drive decision making from data.
Q: What are your thoughts on how data scientists and employees can work more productively together?
A: I would suggest that the premise that we’re not working well together should be reframed.
First, there is nothing phenomenologically new that the term “data science” has introduced other than the fact that we are formalizing methods that you learn from mathematics and applying them to problems that are important to an organization’s mission. That’s all it is. Nothing has changed from that perspective. Sure, the tools have changed and evolved, but the same methods that are used in statistical inference and linear models have been around for almost 200 years.
The second point is this: Data science is not and should not exist if there is not a joint understanding between business and the data science practitioner to work together to serve our customers – the American people. You need to really interrogate the specific questions you want to answer, and (make sure) there’s a justifiable reason to the business that the use of data and doing analysis is much more beneficial than gut instinct or political expediency.
Q: From your experience, and in the spirit of working more closely together, how can we create better working relationships between data scientists and employees at CMS who use data?
A: One way to make our organization more efficient is to have accountability and mandated metrics defined in which working groups work together on data problems.
If you are working on a project, we should strive to work more efficiently by allocating people based on their strengths and expertise – specifically as it pertains to achieving a project deliverable – regardless of where they are in the organization or their tenure at CMS.
At the same time, we should rely less on contracting and more on cultivating, hiring, and retaining people with specialized skills that lend themselves to “data science,” clinical practice and public health (both policy and biostatistics).
Q: What can leadership do to move our organization closer to that ideal?
A: Senior directors and leadership really need to focus on ironing out the problem, developing measures of accountability, cutting out middlemen, and not assuming that data scientists can automatically come in and solve an ill-defined problem.
We should focus on implementing solutions that are actionable and implementable and directly affect CMS’s strategy and objectives. These are some of the things that leadership should really think about when they assign strategy or decide what the organization is going to be working on for the next three, six and 12 month intervals.
We should also have more feedback loops for performance and growth and development plans that are not rooted in OKRs that are not tied to a specific measure or metric for success. If there is a need to actually better understand a problem, then research and time should be spent with more experienced candidates of the “data science” spectrum.
Q: So what should our focus really be on here?
A: I have only been here since February, so I think it’s a bit of a stretch to ask me what we should focus on – particularly if every working group with CMS has a deeper understanding of their purpose, mission and stakeholders.
What I can give an example of, based on a few projects that I have worked on, is that the real challenge here is answering the following: Can we concretely change policy or use analysis to actually inform policy making or legal recourse? And if so, how do we best do it?
Our collective time should be spent on identifying the problem, coming up with a systematic way of designing experiments, identifying the proper data sources that can answer questions within a problem domain, perhaps implementing a model for inference or prediction or even for characterization of the problem, acting on those results and making sure everyone stays abreast of updates and any changes that affect their work. Most importantly, we need to have accountability/feedback loops for both measures for successes and failures. Without that, data science is basically useless.
Q: Have you worked in environments like this?
A: I have never worked in federal service where an organization has so many policy initiatives, expenditures, and serves a need – not a want. However, I have been part of everything from startups to large multinational companies with teams that span several continents. The core attribute that defined their success and existence was premised on how well we translated the efficacy of every product and feature we built into monetization or some other business value.
A big part of our systems of accountability was a laser-focus on well designed data collection systems oriented to measuring business value. Therefore, our experiments and measurement studies had to be very well-designed with an interdisciplinary team spanning engineers, domain experts – such as marketing and sales professionals - and research scientists with skills that were applicable to the “data sciences.”
That being said, this approach does not just have to apply to commercial and consumer-facing products; we can do this within CMS. We roll out countless policies and rules and plenty of new mandates to states that affect millions of beneficiaries and countless healthcare professionals. We can approach policy and practice with the same eye towards data-driven decision-making.