Building Smart: How the Cloud Structures Data for AI
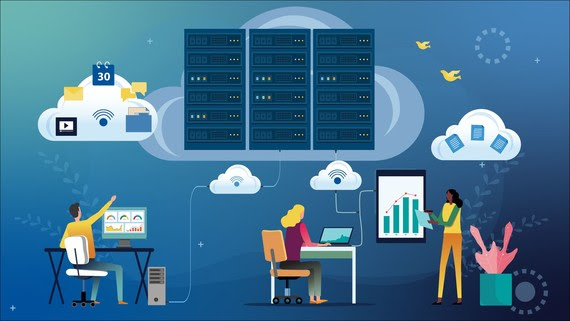
Putting data in the cloud is kind of like building castles in the sky. Except that you can really do it. And you should.
Of the many ways that cloud computing enhances our ability to innovate at OIT, one of the most important is helping us create new data architectures. In fact, cloud technology will pave the way for an AI-enabled data ecosystem at CMS. How exactly does the cloud optimize data for AI initiatives?
1. Power
AI technologies, such as machine learning (ML) and natural language processing (NLP), require extensive amounts of data and processing. The more data there is to “train” an algorithm, the better the algorithm will be able to do its job. Processing all this data requires significant computer power. Because cloud services are not limited by the computer hardware that can fit on a physical premise, they offer the power necessary to adequately train algorithms.
2. Architecture
Traditional database technology links the two capabilities of “storage and compute.” The cloud lets us disconnect data processing from data storage. Without storage and compute yoked together, we can build new scalable architectures and create new workflows. For example, the cloud enables a broad spectrum of new massively parallel processing (MPP) technologies, meaning that multiple processors can work on different parts of a program at the same time. These technologies are the basis of big-data and AI processing.
Additionally, by storing data separate from compute on the cloud, we can minimize or eliminate the unnecessary movement of large data sets, manipulating them without altering the source data. We can also save costs by decoupling computing resources from storage resources, and we can scale more easily. With more power and better architecture, data scientists can also quickly conduct proofs of concept. Most importantly, they can create new data platforms that are more conducive to AI technologies.
3. Flexibility
These new architectures introduce more flexibility into a data system. This is crucial because the AI ecosystem uses algorithms that: a) come from a variety of different sources, including open source, academic institutions, and large corporations, and b) evolve rapidly. Segregation of storage and compute patterns allows one to apply and adapt multiple technologies quickly. Therefore, the cloud offers the adaptability necessary for addressing a broad swath of AI use cases and experimenting freely.
Chief Technology Officer George Linares is seizing on that flexibility by spearheading a new, decentralized data architecture called a data mesh. "The CMS Enterprise Data Lake (Data Mesh) Initiative will empower everybody at CMS who deals with data," Linares explains. "In other words, it will empower everybody.”
Manjunath Salimani, architect of the data mesh, says that, “It democratizes data infrastructures with low friction for data scientists to access data with the appropriate governance, and provides data in a way that is optimal for AI/ML workloads.”
The cloud doesn’t just provide space for parking our data. It opens up entirely new possibilities for organizing it, sharing it, and ultimately, deriving insights from it.