What's New in Data Science?
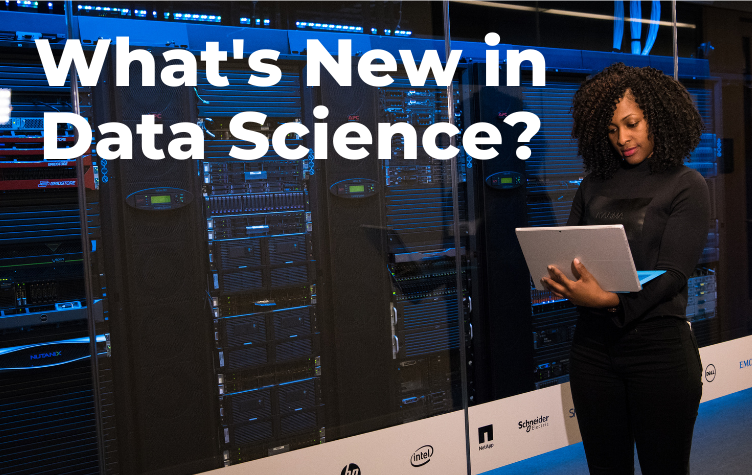
In a 2012 Harvard Business Review article, Thomas H. Davenport and D.J. Patil dubbed data scientist “the sexiest job of the 21st century.” At the time, data science was a completely new profession. The term had only been coined in 2008 by Patil, who would eventually become the first U.S. Chief Data Scientist.
Fast forward 10 years, and the field of data science continues to evolve at an extremely rapid pace. This evolution is fueled by new practitioners, new data, and new technologies. As 2022 kicks into high gear, we take a look at some of the latest trends shaping data science.
Convergence
AI, Internet of Things, Cloud Computing, 5G: all of these technological innovations will increasingly overlap. By playing together, these technologies enable some of the capabilities mentioned in the rest of this list, such as AI automation, small data analysis, and edge computing.
New, more complex capabilities will lead to new methodologies. The term “XOps” might begin to replace the term, “DataOps.” DataOps infuses the lifecycle of data analysis into DevOps, which in turn combines Agile software engineering and IT processes. XOps adds practices devised for the development of AI to DataOps .
Python
Currently, the programming language most used for data science applications is R (because it is great for data visualization), but Python’s ascendency is inevitable. It is expected to overtake R within the next few years.
Python is free, open source, and it has a simple, easy-to-use syntax. That means even if you don’t have a background in software engineering, it’s not too hard to learn. There are also several existing libraries for use in data science applications, including Machine Learning.
Python is flexible, so it can be adapted or integrated for different types of tasks. It scales well, allowing for large teams of developers to build applications together. Best of all, it’s named after the British comedy troupe Monty Python.
Computing on the Edge
While cloud computing gives data scientists the ability to store and analyze large sets of data in a central location, there are increasing opportunities for data to be processed at the “edge” of the network, where it is collected. This data may be housed in web-connected devices such as those found in smart homes, electrical grids, or healthcare settings where medical instruments, sensors, or computers log large quantities of patient data.
Filtering and processing data at the edge reduces the constraints of latency (a measure of delay), bandwidth, and congestion. It can also alleviate compliance and security concerns because data is not traveling to third-party infrastructure. Lastly, it allows for real-time data so that organizations can interact with customers as they are using products and services.
Small Data
Such real-time insights are often associated with the concept of “small data.” For much of its existence, data science has been seen as the answer to Big Data. The ability to manipulate enormous amounts of data has led to excellent predictive, descriptive, and prescriptive analytics. Generally, the bigger and better the data set, the better the insights. Now, with the help of AI, data scientists are learning how to draw actionable information from smaller, contextualized data.
Automation
You might see AI Automation (AA) referred to as “augmented analytics.” No matter the terminology, the idea is that organizations can apply prepackaged AI models – including machine learning and natural language processing – to analyze their data. These models complete steps in the data lifecycle such as cleaning, labeling, and creating visualizations. AA gives nonspecialists the ability to extract insights from data. It also saves organizations time and resources.
Responsible AI
When it comes to data, privacy and security are critical concerns. Security breaches at both public (e.g. U.S. Office of Personnel Management, 2015) and private (e.g. Facebook, 2019) institutions regularly remind us about threats to our personal data.
AI also presents new ethical challenges. We should be careful to equally distribute the benefits and risks of new technologies, eliminate bias, and to regulate against malicious use. As algorithms become more ubiquitous and more powerful, it’s important to ensure that they are deployed responsibly.
In September 2021, HHS released the Trustworthy AI Playbook. This guide delineates six principles of Responsible AI, based on Executive Order 13960, “Promoting the Use of Trustworthy Artificial Intelligence in the Federal Government,” and Office of Management and Budget Memorandum M-21-06, “Guidance for Regulation of Artificial Intelligence Applications.” The principles are:
- Fair/impartial - to ensure equitable application across all participants
- Transparent/explainable - so relevant individuals understand how their data is being used and how AI systems make decisions
- Responsible/accountable - including governance and who is responsible for an AI solution
- Safe/secure - to protect from risks, including Cyber, that may cause physical or digital harm
- Privacy - so data is not used beyond its intended use and uses are approved by the data owner
- Robust/reliable - resulting in accurate and reliable outputs consistent with the original design